Our arxiv paper "Lotus Diffusion-based Visual Foundation Model for High-quality Dense Prediction" is getting noticed

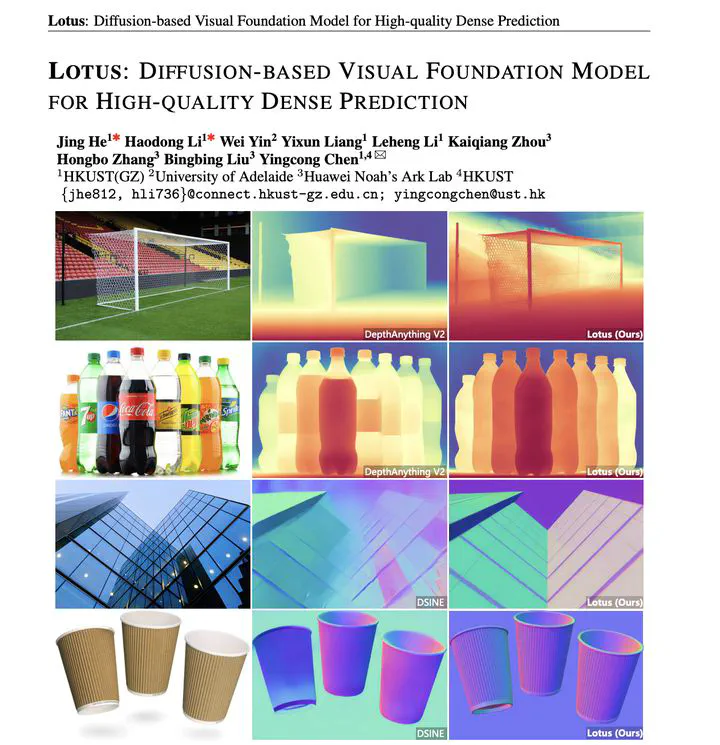
We are excited to introduce Lotus, our newly developed diffusion-based visual foundation model that marks a significant leap forward in dense geometry prediction. Lotus achieves state-of-the-art performance in key tasks such as zero-shot depth and surface normal estimation, setting a new benchmark in computer vision.
Dense prediction tasks, such as zero-shot depth and surface normal estimation, require precise pixel-level predictions, making them challenging to optimize with traditional methods. Our work delves deeply into the adaptation of diffusion models, which have primarily been used for image generation, to dense prediction tasks. Through our comprehensive analysis, we have identified and resolved several key challenges that arise when applying these models to dense prediction scenarios.
Key Findings:
Noise Prediction vs. Direct Annotation Prediction: We discovered that the standard parameterization used in diffusion models—predicting noise—introduces significant variance, which propagates through the iterative process and degrades prediction quality. Instead, we propose training Lotus to directly predict annotations, which mitigates this variance and results in more stable and accurate outputs.
Single-Step Diffusion Process: Traditional diffusion models rely on multi-step processes, which are not only computationally intensive but also prone to error accumulation. Our experiments show that a single-step diffusion formulation is not only simpler but also leads to better performance, especially when training data is limited. This finding significantly improves the efficiency of Lotus, making it hundreds of times faster than existing models.
Detail Preserver: While adapting diffusion models for dense prediction, we observed that models tend to lose their ability to generate fine details, especially in areas with complex structures. To address this, we developed a novel “detail preserver” strategy that ensures high accuracy in detail-rich regions, preserving the fidelity of predictions without compromising efficiency.
By addressing these key challenges and thoroughly validating our design choices, Lotus achieves state-of-the-art (SoTA) performance in zero-shot depth and surface normal estimation across a variety of datasets, with minimal training data. This systematic analysis has allowed us to develop a powerful and efficient model that not only excels in dense prediction tasks but also enables a wide range of applications, including joint estimation and 3D reconstruction from single or multiple views.
This work is selected as the daily paper on Huggng faces upon released.
For more information, please visit EnVision-Research GitHub repository.
Authors:
- Jing He, My student from HKUST(GZ)
- Hao Dong Li, My student from HKUST(GZ)
- Wei Yin, Collaborator from University of Adelaide
- Yixun Liang, My student from HKUST(GZ)
- Leheng Li, My student from HKUST(GZ)
- Kaiqiang Zhou, Collaborator from Huawei
- Hongbo Zhang, Collaborator from Huawei
- Bingbing Liu, Collaborator from Huawei
- Ying-Cong Chen, HKUST(GZ)