Our Paper “Ref-NeuS” Nominated for Best Paper at ICCV 2023

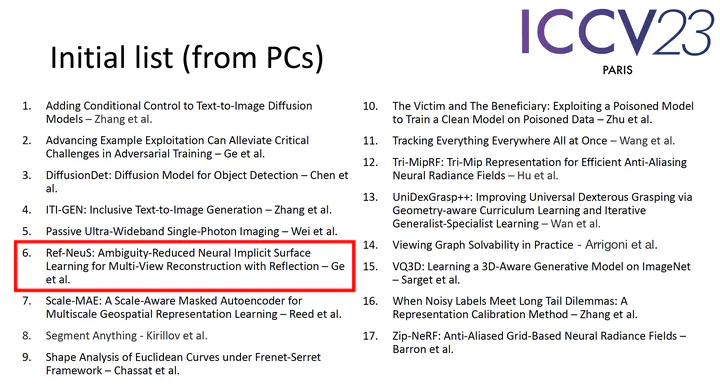
I am thrilled to share the exciting news that our paper, titled “Ref-NeuS: Ambiguity-Reduced Neural Implicit Surface Learning for Multi-View Reconstruction with Reflection,” has been nominated for the Best Paper Award at the prestigious ICCV 2023 conference. This nomination is a testament to the hard work and innovative research conducted by our team. Below is a brief overview of our paper and its contributions.
Overview
Abstract
Neural implicit surface learning has shown significant progress in multi-view 3D reconstruction, where an object is represented by multilayer perceptrons providing continuous implicit surface representation and view-dependent radiance. However, existing methods struggle to accurately reconstruct reflective surfaces, leading to severe ambiguity. Our proposed model, Ref-NeuS, addresses this issue by attenuating the importance of reflective surfaces through a novel approach.
Introduction
3D reconstruction is essential in computer vision, serving as the foundation for fields like computer-aided design, animation, and virtual reality. Image-based 3D reconstruction, which recovers 3D structures from 2D images, is particularly challenging. Traditional methods often require cumbersome multi-step pipelines. Our approach leverages neural implicit surface learning for end-to-end and unsupervised training, achieving high-quality reconstruction even on reflective surfaces.
Key Contributions
- Reflection-Aware Photometric Loss: We introduce a reflection-aware photometric loss that reduces the influence of reflective surfaces. This is achieved by modeling the rendered color as a Gaussian distribution, where the reflection score represents the variance.
- Reflection Score Estimation: We utilize an anomaly detector to estimate an explicit reflection score with multi-view context guidance to localize reflective surfaces accurately.
- High-Quality Surface Reconstruction: Our model significantly outperforms state-of-the-art methods in reconstructing reflective surfaces, providing more accurate surface geometry, normals, and rendering realism.
Results
Our extensive experiments demonstrate that Ref-NeuS achieves high-quality surface reconstruction on reflective surfaces, outperforming other methods by a large margin. The model is also competitive on general surfaces, showing its robustness and versatility.
Conclusion
Being nominated for the Best Paper Award at ICCV 2023 is a great honor for our team. It acknowledges our efforts to advance the field of 3D reconstruction with innovative solutions that address long-standing challenges. We look forward to presenting our work at the conference and engaging with the community to further this exciting research.
For more details, you can access the full paper here.
Authors:
- Wenhang Ge, My student from HKUST(GZ)
- Tao Hu, Collaborator from SmartMore and CUHK
- Haoyu Zhao, My student from HKUST(GZ)
- Shu Liu, Collaborator from SmartMore
- Ying-Cong Chen, HKUST(GZ)